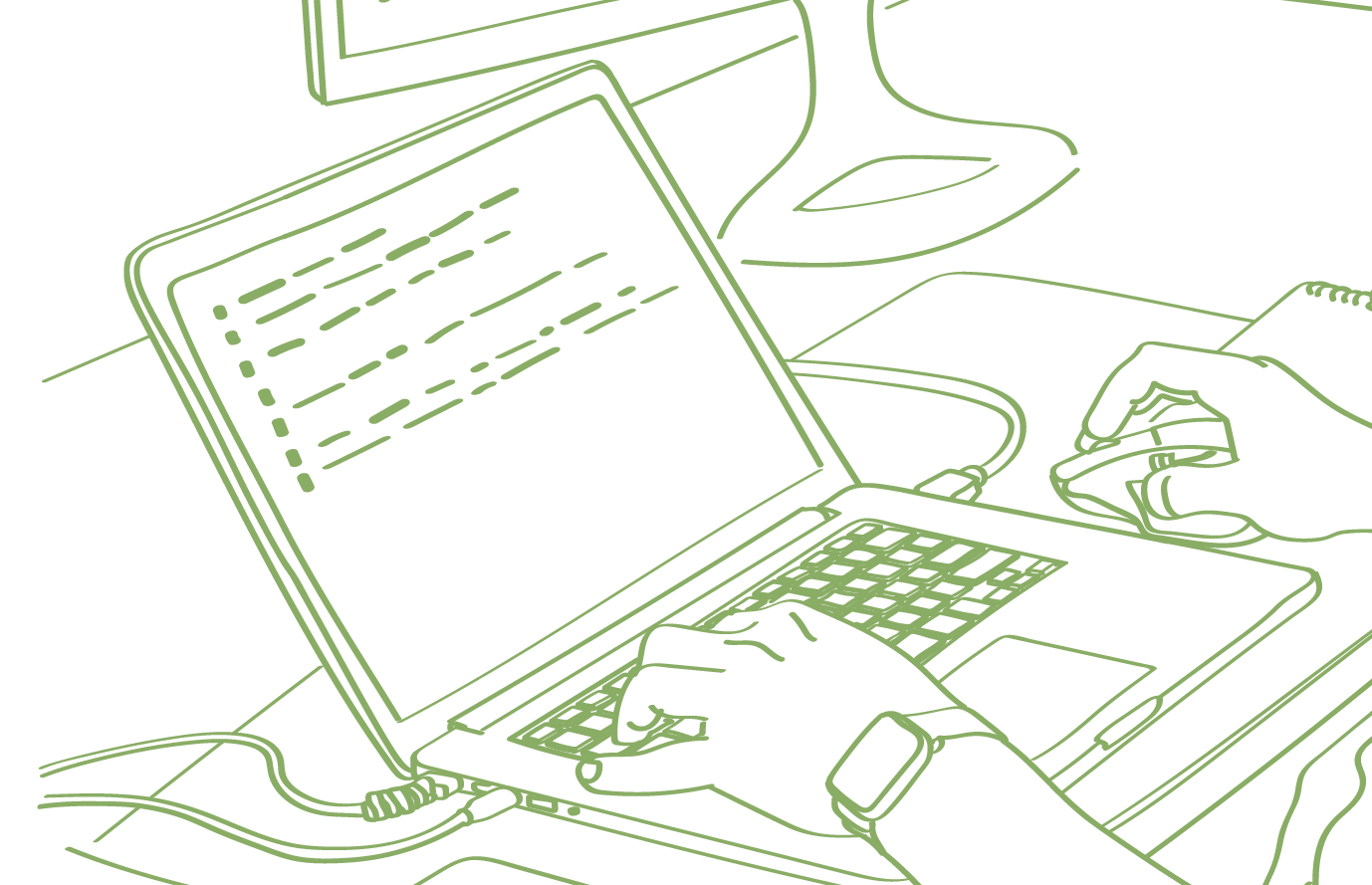
Carbon Re brings together experts in machine learning and cement to build models that help cement producers gain greater oversight of their plants, lowering their carbon emissions while maintaining the cement quality they require. However, the journey doesn’t end when our models are deployed to our customers’ plants: it is crucial that the machine learning team at Carbon Re is able to continuously monitor the models’ performance and their effect on the plants’ operation. Our internal monitoring platform serves as the central nervous system of our models. It provides a real-time dashboard that tracks the accuracy and reliability of our models, as well as the state of the plant, flagging unusual behaviour. This allows the team to quickly analyse and react to any issues.
Soft sensors
One of the key solutions offered by Carbon Re is our soft sensor modelling: we train models to make higher frequency predictions for measurements that are traditionally monitored at a lower frequency. For example, measurements of free lime (a key quality metric for cement) are typically only available every few hours. Our free lime soft sensors generate predictions every 15 minutes, effectively filling the gaps between real measurements and enabling the operators to have a more consistent view over the plant, ensuring better operational stability.
Our monitoring dashboard helps us quickly investigate issues with these models. For example, when the platform started flagging the predictions of one of our soft sensors as being outside the expected range, the team was able to quickly drill down on the issue. We established that the root cause was that the plant in question was operating at a significantly lower throughput than usual, as it was gradually being shut down for maintenance. Since our model was not trained to operate in this type of plant condition, the behaviour was expected.
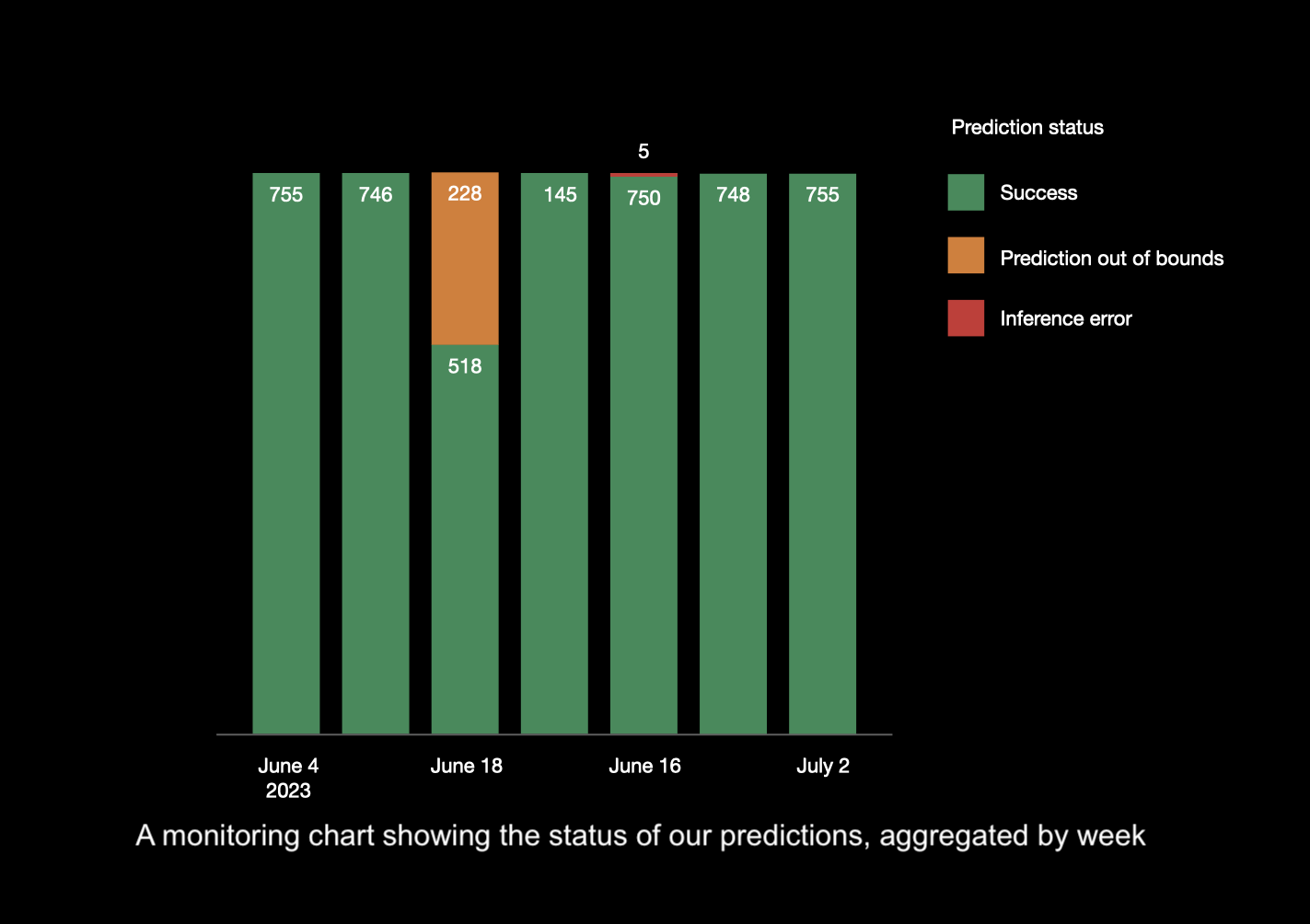
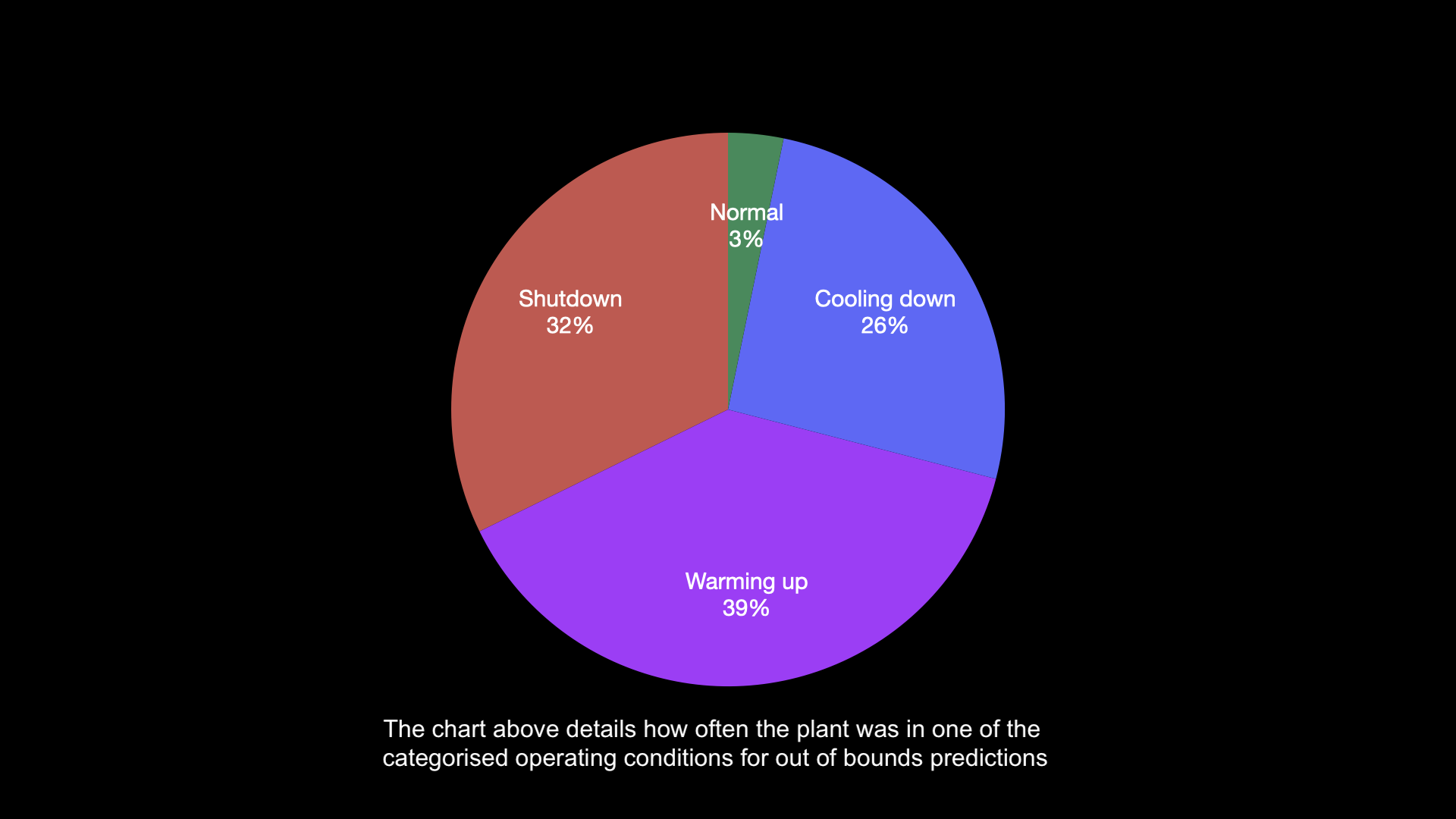
The platform also alerts us when the soft sensor’s predictions start to deviate significantly from the actual measurements, potentially indicating that the plant’s operational dynamics have shifted (for example due to maintenance, or to equipment wearing out). This would be a cue for the team to retrain the models to match the new conditions.
Recommendations
One of our other main offerings is our dynamic recommendation system: based on the real-time conditions within the plant, it suggests adjustments to key control parameters and forecasts the expected outcomes of implementing those adjustments. Once these models are deployed, our monitoring platform allows us to monitor some key aspects:
- Operator Engagement: Plant operators receive the recommendations on our platform, and can choose to accept or bypass any given recommendation, with the option of giving a reason in the latter case. If our monitoring platform flags an unusually high number of rejected recommendations, we can investigate the system to find any issues, potentially reaching out to our client to discuss necessary improvements
- Prediction Accuracy: When the recommendations are accepted, it’s crucial to check whether the outcomes match our model’s predictions. The monitoring platform allows us to track the model’s accuracy, just as it does for the soft sensors, ensuring we can quickly detect and react to any issues
Overall, our monitoring platform serves as a fundamental feedback mechanism. By tracking how our models perform in the field, we can ensure that our soft sensor and recommendation systems keep delivering value to our clients, pushing forward on our commitment to decarbonise heavy industries.