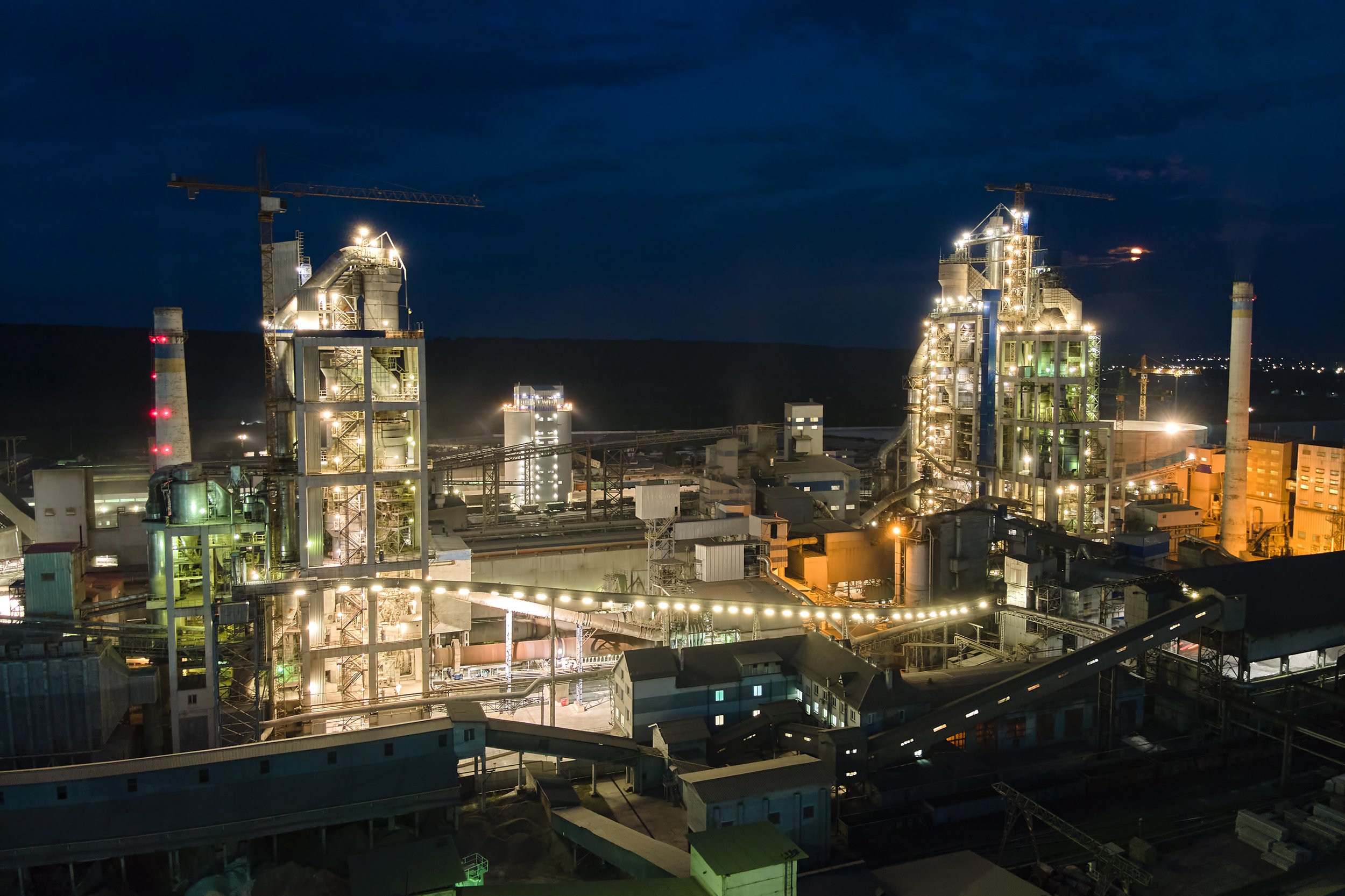
Cement production is a complex process at the best of times – requiring constant balancing of a whole host of different parameters that affect cost, quality and reliability. Add to the mix escalating fuel prices and the increasing emphasis on CO2 reduction targets and it’s clear the challenge is now greater than ever.
Plant operators are skilled at managing these conflicting requirements, supported by a range of expert systems, predictive process controls and automation systems. These software solutions can all maintain operations within desired limits. But they lack the dynamic qualities that are vital to deal with changing plant parameters or improve performance beyond a narrow operating window.
The settings for all these traditional tools are fixed when the systems are set up – meaning they inevitably drift over time as things change. The introduction of different materials or fuel, for example, will affect the accuracy of the systems unless the parameters are regularly updated – a human intervention that is time consuming and expensive.
In contrast to this inflexible approach, artificial intelligence (AI) is now offering a real-time approach to the challenge that is as unique as each individual cement production plant. Optimisation becomes a constant process, not a one-off adjustment in control settings – unlocking valuable business benefits.
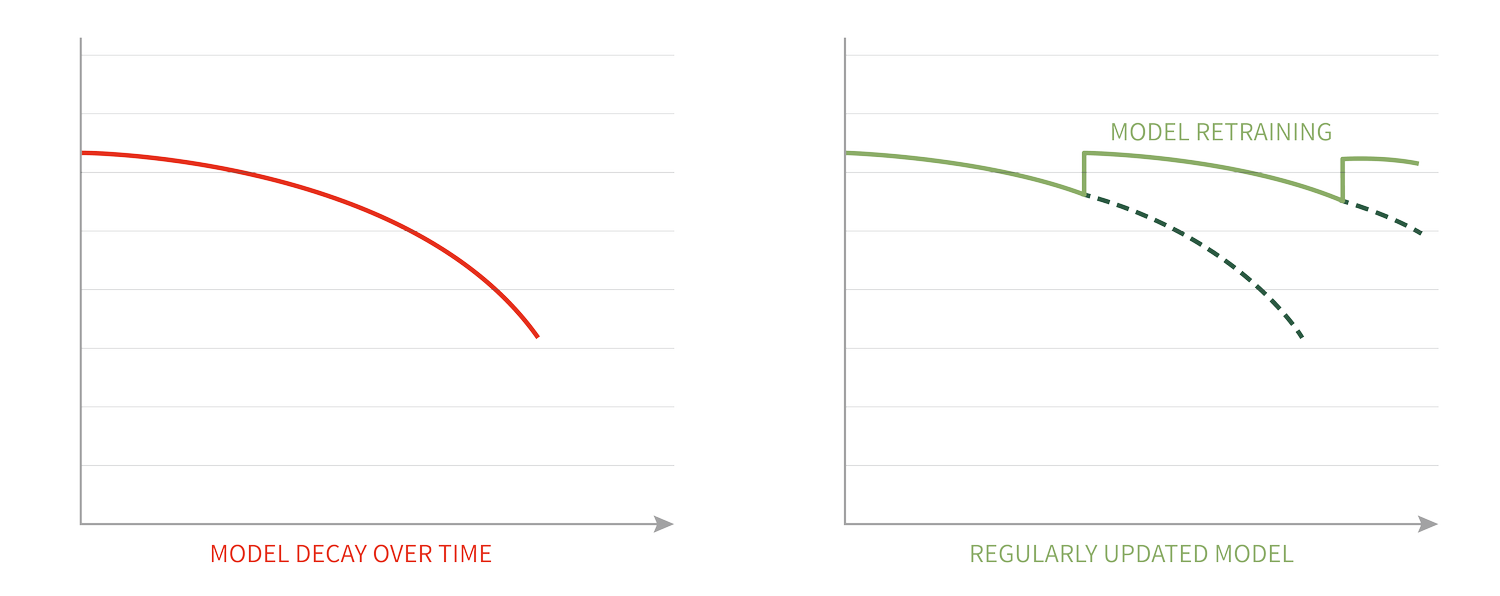
That’s why here at Carbon Re we have developed our unique Delta Zero software platform, powered by AI and advanced machine learning. Our technology creates a digital twin of an individual plant by analysing the sensor data gathered on a minute-by-minute basis by distributed control systems. This digital model then delivers an accurate representation of the real-world performance in that specific plant.
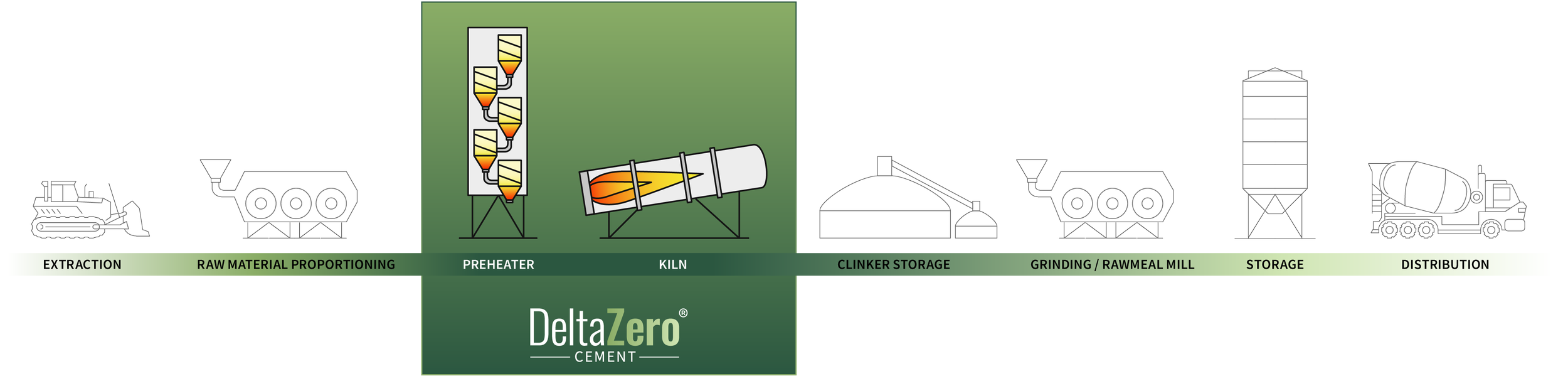
Carbon Re Cement focuses on the critical pre-heater and kiln process stages that account for all thermal fuel use and emissions to optimise fuel use and reduce carbon emissions.
The AI system learns by finding relationships between the plant operating data – in the same way a human does. The difference is that advances in computing and high-speed processors mean the AI system can experience the equivalent of 100 years of cement plant operation in simulation during a day’s training.
As a result, Carbon Re can help even the most experienced operator in ways that are beyond any traditional expert system. It can predict output clinker quality from feed rate, fuel choices and other kiln performance raw material quality parameters, for example. The predictions are near real-time and in advance of the quality measurements the plant conducts. Output clinker quality can then be understood immediately when the input control decisions are made – avoiding the time lag before clinker quality tests can be conducted on the output of the kiln.
Its ability to operate as a standalone system reduces the set-up time of the platform to just 2-4 months while the plant data history is connected and the AI models are trained. That’s a radical reduction compared with the typical 3-5 years it takes to implement an expert system. The Carbon Re platform requires little systems integration, hardware investment or plant operator training.
The Carbon Re platform has been designed as a human-in-the-loop solution, with a simplified dashboard to highlight recommended settings for the plant operator. But it can also work alongside expert systems, predictive process controls and automation systems to augment their value to the business.
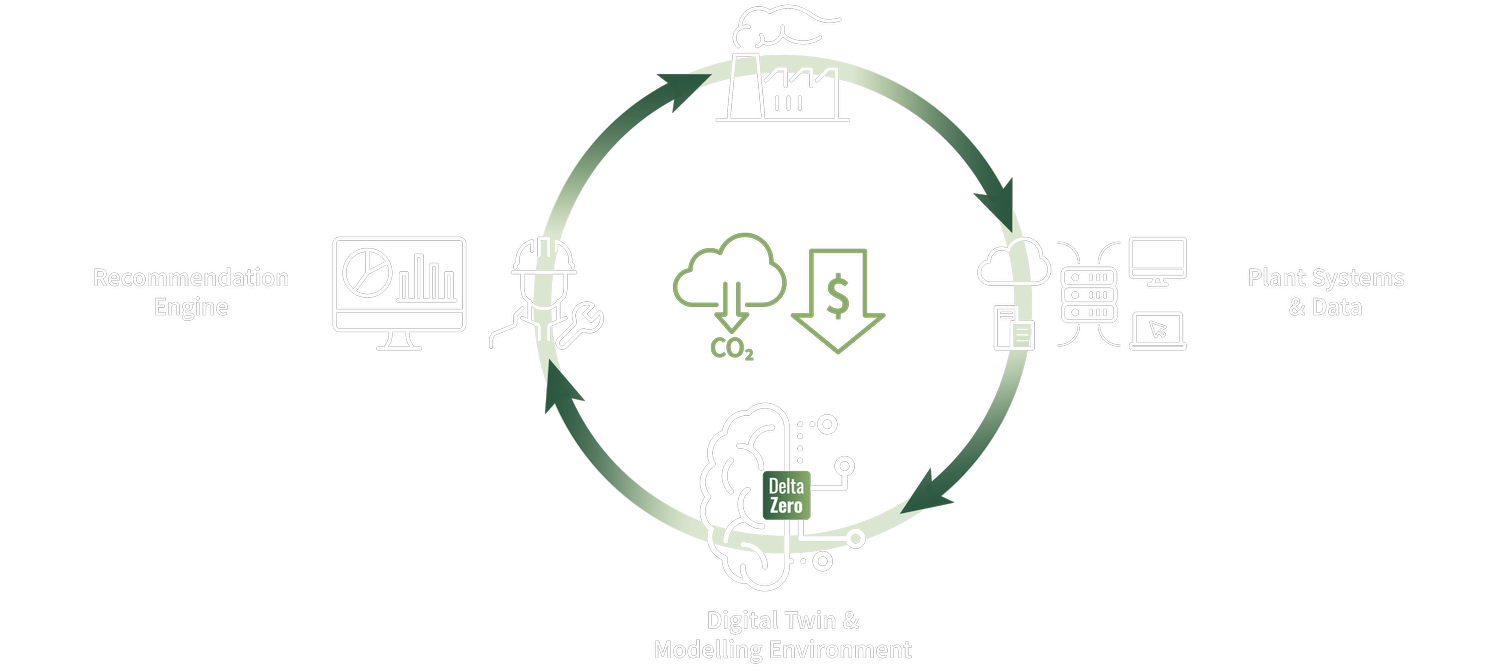
When combined with automation systems, Carbon Re can suggest temperature levels to maintain for optimum performance, for example, with greater accuracy than predictive process controls. Our AI system can also optimise the parameters – or target settings – of an expert system. And it can optimise the rules used by predictive process controls.
Carbon Re can enable cement plant operators to achieve up to a 10% reduction in fuel consumption, primarily by optimising excess air ratios to minimise energy loss to the stack and tailoring heat input dependent on the raw materials and fuel choices. This requires regular minor adjustments of parameters by a few percentage points. As the input parameters are constantly changing, so are the recommendations – something that could not be done in real time by traditional systems.
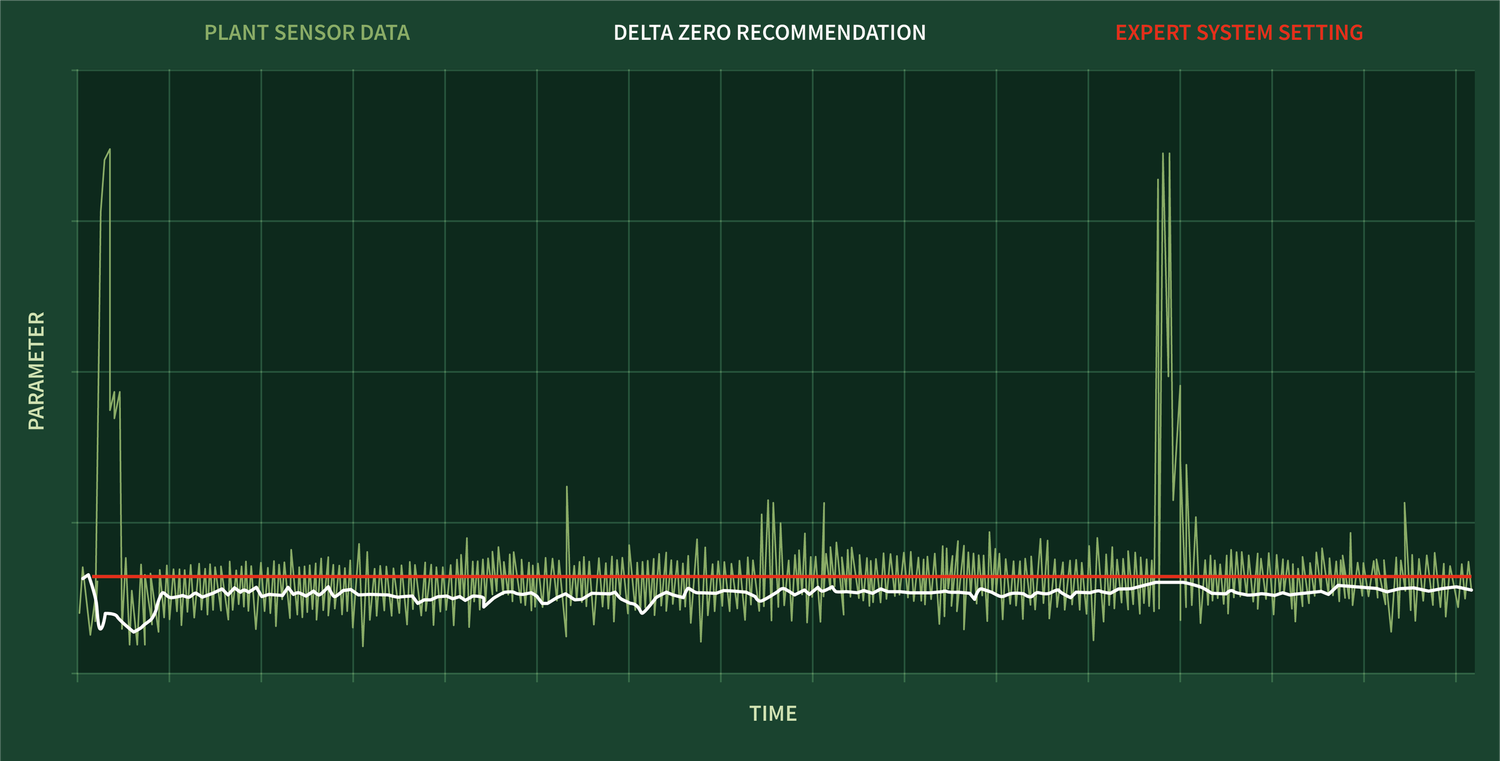
Fuel-derived carbon emissions can also be cut by up to 20% with the help of the platform. It offers a way to navigate the switch away from fossil fuels to a blend of alternative fuels such as biomass, industrial waste, domestic waste or dried sewage.
The composition of alternative fuels affects the production process in different ways – from moisture levels to volatile contents and particulate size. All these different interactions add complexity to decision making on plant control parameters. AI can simulate the impact of potential fuel mix combinations and learn the relationship between performance and blending of different alternative fuels. This level of sophistication is beyond the reach of legacy expert systems, predictive process controls or automation systems.
Advances in AI and machine learning are already transforming industries ranging from finance to healthcare. Now it’s the turn of the cement industry to reap the benefits of harnessing this new generation of technology to tackle some of its greatest challenges.