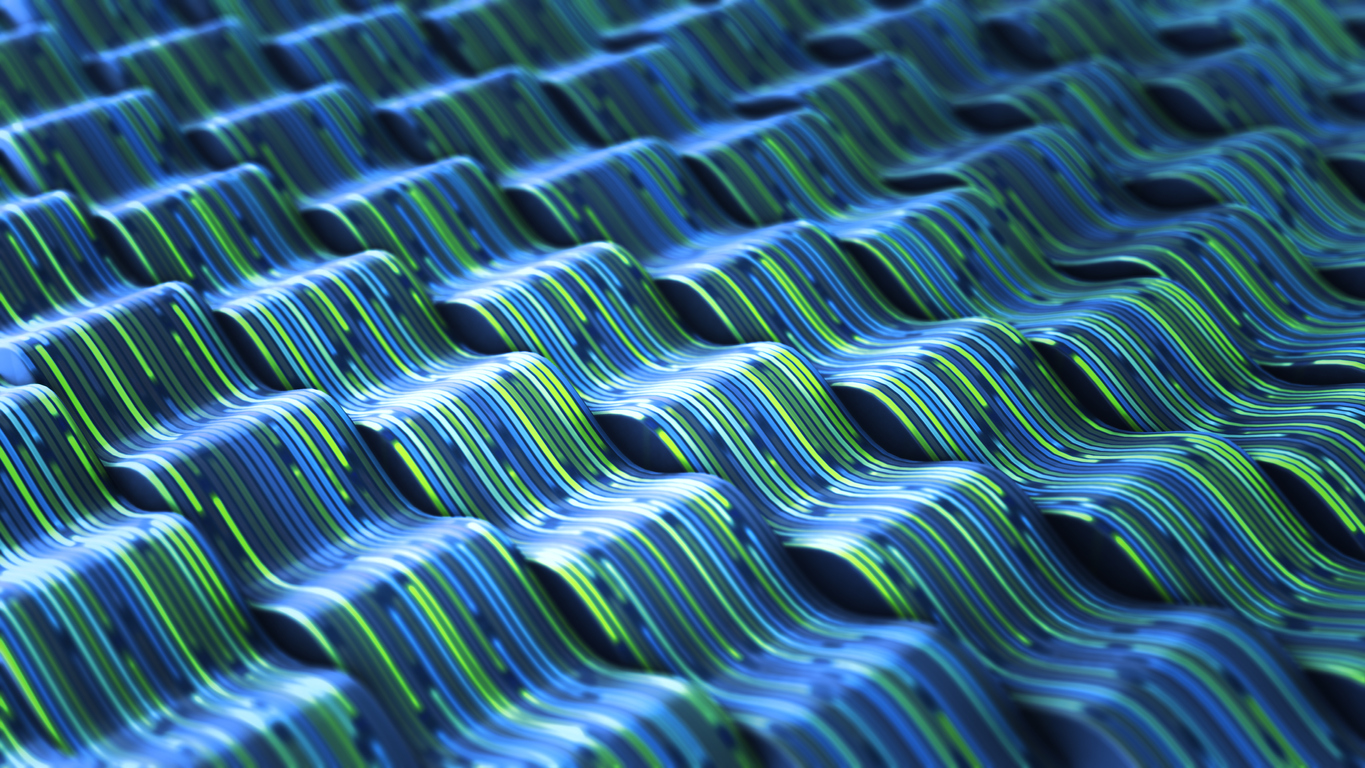
In December 2023, over fifteen thousand researchers and engineers traveled to New Orleans to attend NeurIPS: the biggest AI conference of the year. This year again, the dominant presence of tech companies reflects the shift in focus from academia to industry that we see happening in the AI research community.
Over a year after the general release of ChatGPT (the fastest consumer application to reach 100 million users) put AI at the centre of public discourse, the biggest-ever attendance at NeurIPS reflects the extent of global interest in the advancement and use of AI in public life. The technology behind ChatGPT, Large Language Models (LLMs), can now be found everywhere, and this was no exception at NeurIPS.
LLMs permeated all the poster sessions, where they were used to prove mathematical theorems, do legal reasoning and even to predict the next value in a time series. There is an enormous effervescence around the field. Excitement is everywhere.
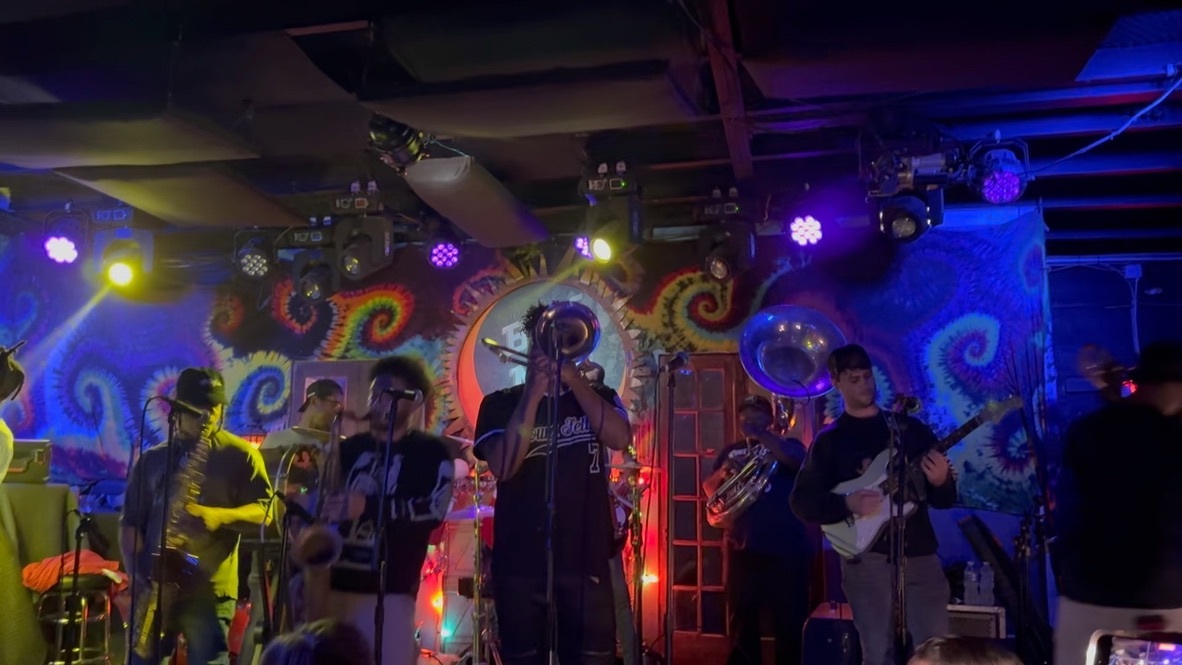
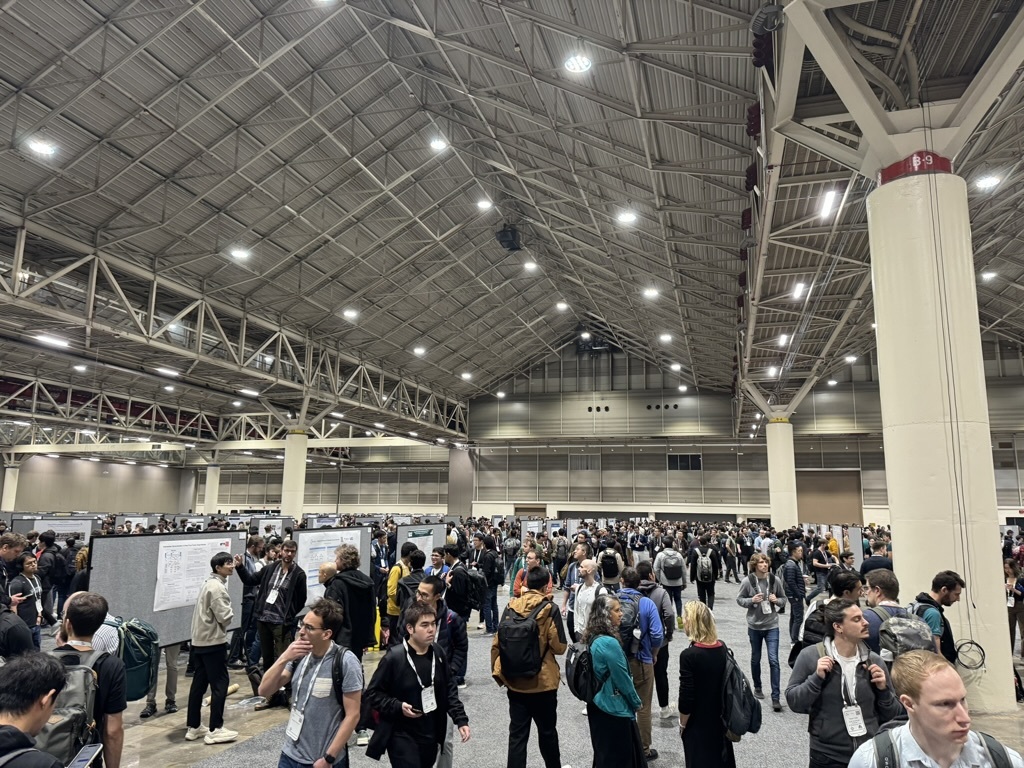
New Orleans; the birthplace of Jazz. Meeting AI “celebrities” in Jazz bars was quite an experience (left). The first poster session at the conference (right).
The AI 4 Climate Community
Despite the pervasive enthusiasm, I have little interest in LLMs, and we don’t use them for our modelling at Carbon Re.
The conference organisers provide an app that can be used to contact participants, which I used to organise meetings with like-minded people who were interested in applying AI to tackle climate change. The AI 4 Climate community is small but driven and I was lucky to meet some brilliant and passionate people in this field. For example, Sungduk Yu a climate researcher from UC Irvine, who wants help from the ML community to help model climate models which are currently extremely complex and computationally expensive.
I was very intrigued with Sungduk’s work, and I had reason to be, it was very novel – he was awarded the Best Dataset Award for his work, ClimSim, a collaboration between 20 institutions and more than 50 researchers. The result is a 40TB high-quality and documented climate dataset – the first of its kind. Climate scientists are constrained by the complexity of their own models which means they struggle to both use, and understand them. Sungduk was pleading with the ML community to attack this problem so that the climate science community could move forward. This talk really resonated with me.
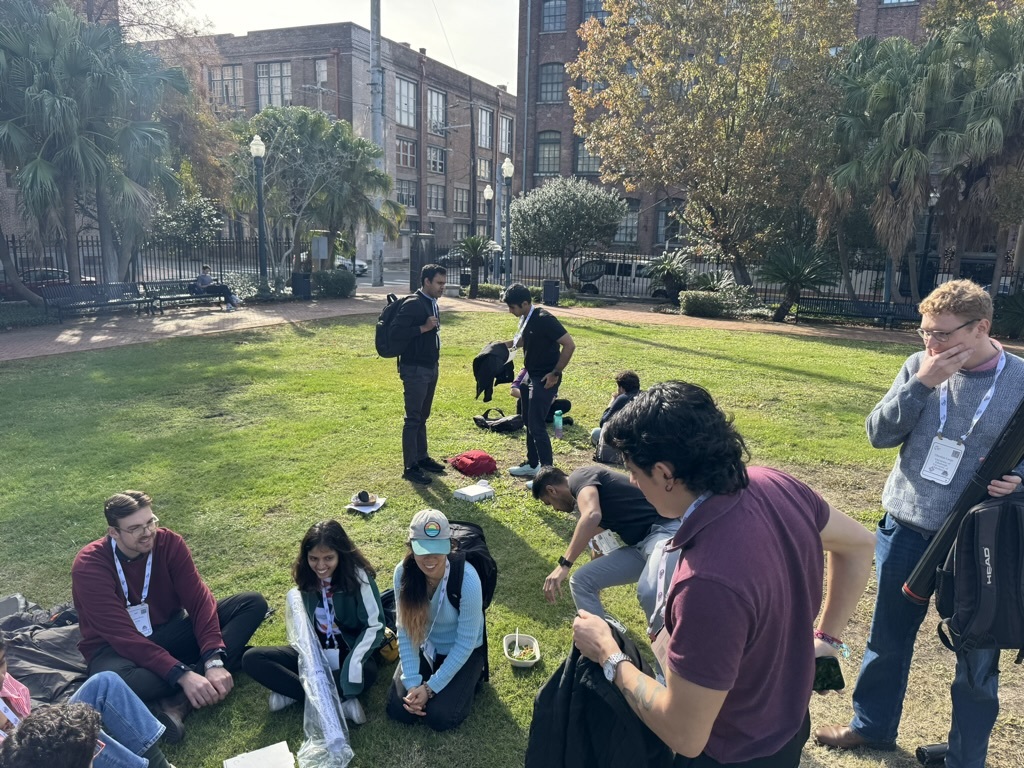
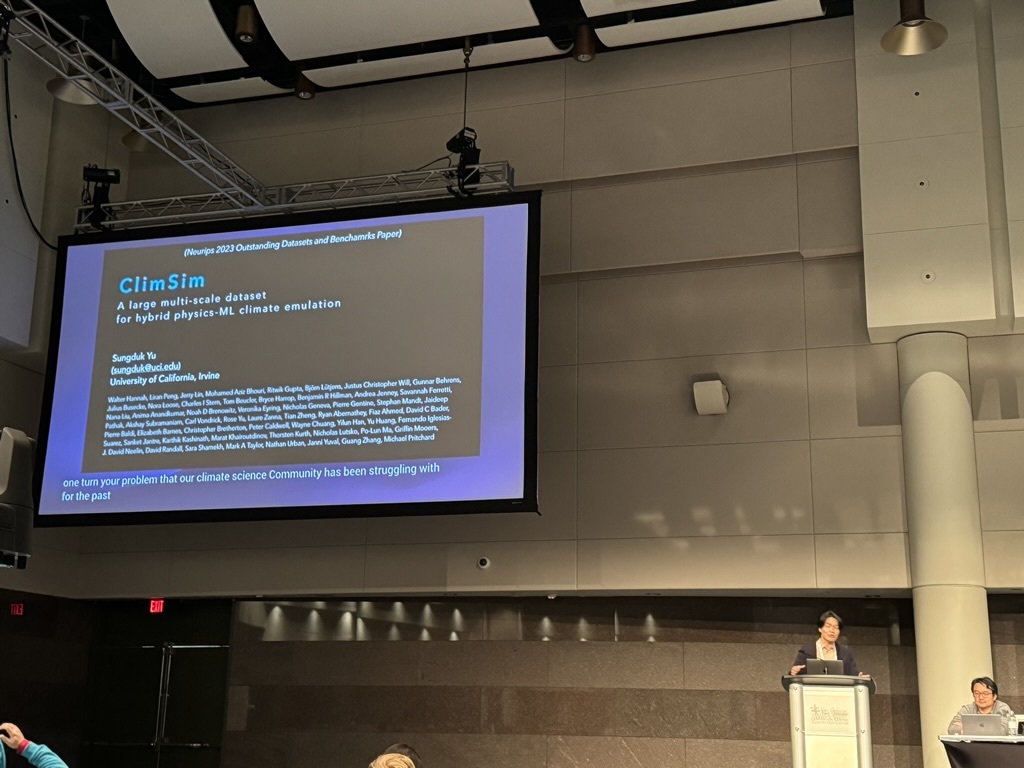
The AI 4 Climate meetup at NeurIPS 2023 (left). Sungduk Yu presenting ClimSim, A large multi-scale dataset for hybrid physics-ML climate emulation. I was very moved by his presentation (right).
Tackling climate change with machine learning
The main focus for my week at NeurIPS was the Tackling Climate Change with Machine Learning workshop organised by Climate Change AI (CCAI), a non-profit organisation at the forefront of the intersection of these fields. Thanks to Carbon Re’s links with CCAI, I met Priya Donti, chair and Co-founder at CCAI as well as assistant professor at MIT and it was great to see that there are truly amazing people working in this field.
I presented our research at the poster session during CCAI’s workshop and was excited to speak to other researchers in our niche, but growing field. While presenting my work to young researchers, I had an impromptu visit from Yoshua Bengio, with whom I had a fantastic conversation about the limitations of the work, and ways to improve it in the future. I was also happy to see many of the people that I had connected with at the two AI for climate meet-ups that I had set up earlier in the week.
Sci-ML
As I was scoured through the posters around the room at the Experimental Design workshop, I found a poster with a title that instantly caught my attention: Sustainable Concrete via Bayesian Optimisation. The author wasn’t there so I sent them a message and within an hour I was talking to Meta Research Scientist Sebastian Ament face to face. He was incredibly nice and all too familiar with the importance of our mission at Carbon Re. His work involves finding ways to build data centres in more sustainable ways, which includes reducing the emissions from the concrete itself. It was very refreshing to (finally) speak to someone about cement and AI without having to start with explaining how cement is made.
At the AI for Accelerated Material Design workshop, I listened to a presentation by Sherrie Yang. She was presenting her work on UniMat, a diffusion model that can find new materials. This was particularly interesting as finding alternatives to Ordinary Portland Cement (OPC) is an important avenue to decarbonising the cement industry.
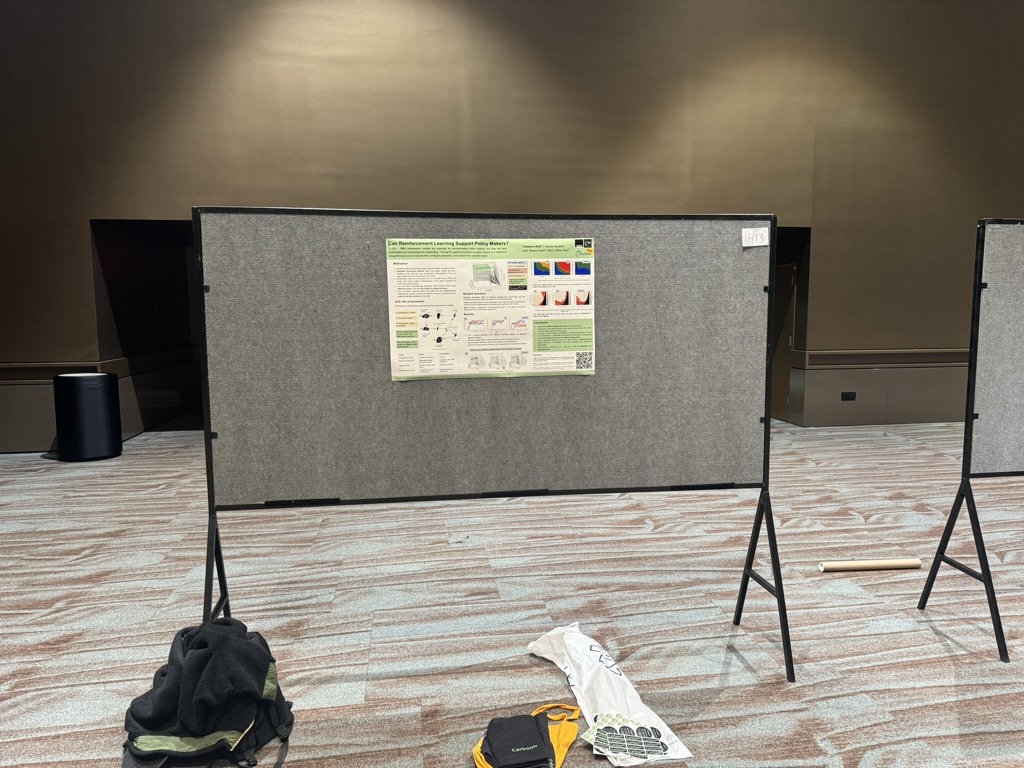
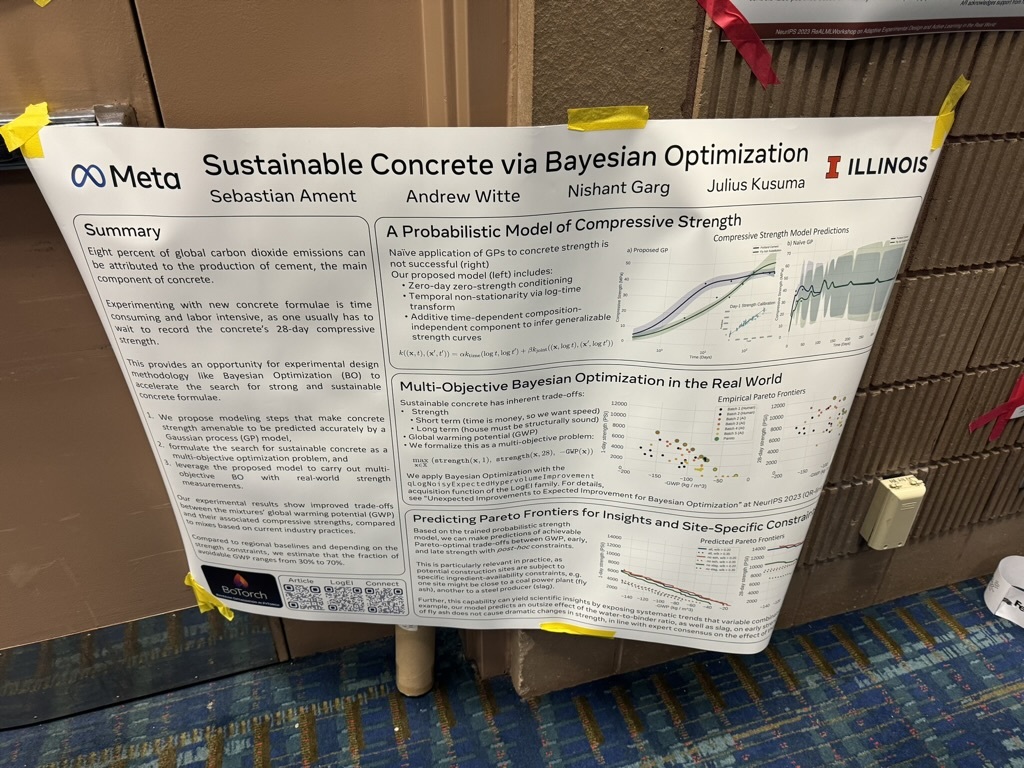
My humble poster setup at the Tackling Climate Change with Machine Learning Workshop. The Carbon Re merch disappeared very quickly! (left). A poster tucked away in the Adaptive Experimental Design and Active Learning in the Real World. The intersection of concrete and AI is pretty damn small (right).
Reflections
NeurIPS is a great example of why scientific conferences are important and necessary for all of us. While there were a lot of researchers who were incredibly interested in what we are doing at Carbon Re, there were also some skeptics, as any good scientist should be. Never before have I been challenged so thoughtfully, in such varied ways, which led to many exciting and eye-opening discussions. Some even led to robust ideas that I can’t wait to test at work in our day to day R&D process.
For my first conference, I’m grateful I was able to attend the largest-ever NeurIPS. I met a huge range of people, many of whom I will certainly meet again – especially given how small the AI-Climate Change Venn diagram is.
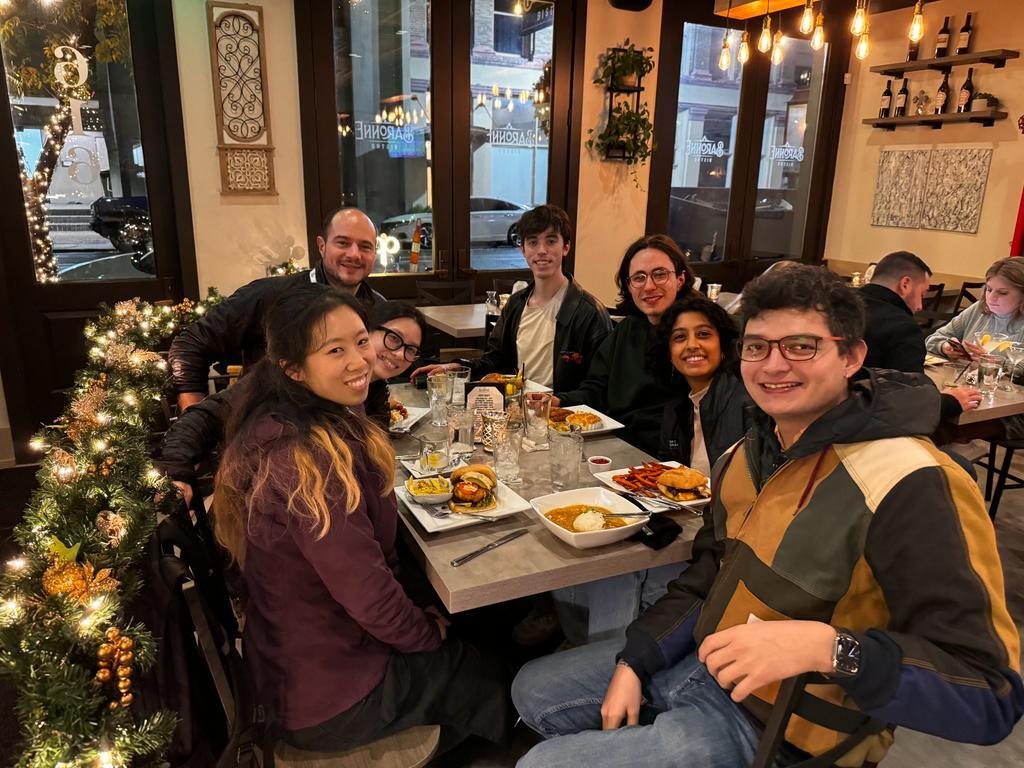
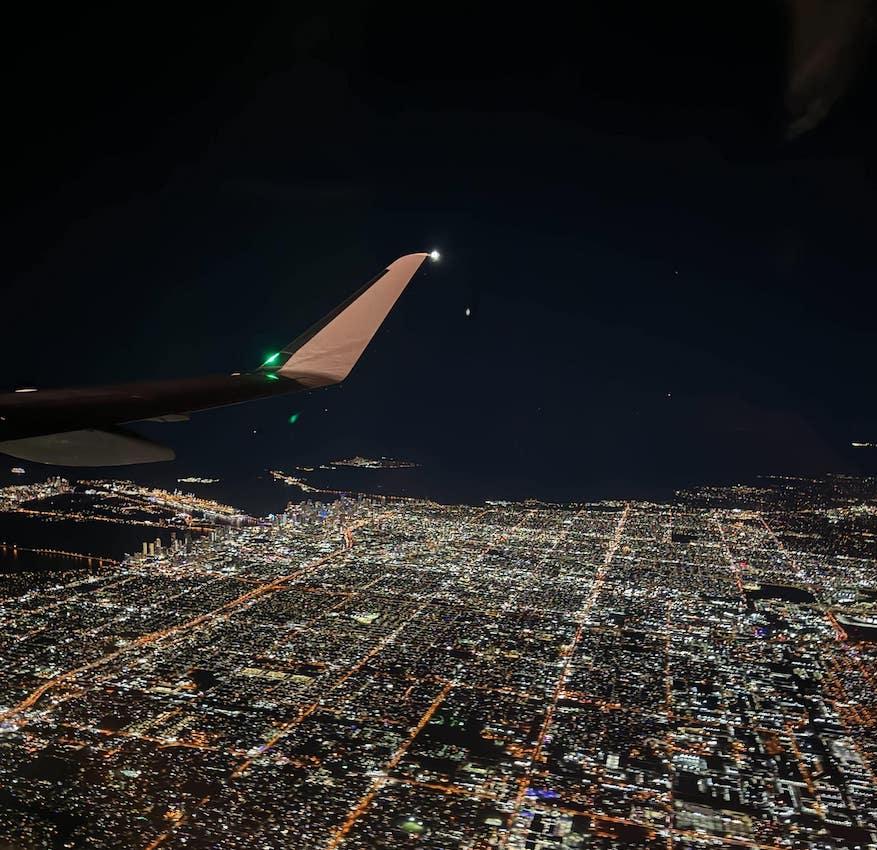
The last dinner at New Orleans with newfound climate-AI friends (left). A last look at the US before the long flight home (left).